May 8
Looked into Dispersy bundle synchronization and Tribler into more detail. Efficient sync using subset bloom filter selection, as well as some good ideas on dealing with challenging networks of various types.
Briefly on large group chats etc
Just to capture briefly ideas from physical notebook. Terse version.
-
Leverage multicast when underlying protocol allows it, i.e. use | topic | message | for sending offers/acks. This assumes you send to many IDs and these are all listening to that topic.
-
Random selection for large group chats. I.e. pick K out of N to offer/ack to, this allows not having quadratic scaling for naive syncs.
-
Log collator idea. f(topic, logs) => topic, one log. Problem: Censorship resistance (proof of data availability~)? No denial of service.
The main idea is to exploit the social connections between people who share similar interests, and use this to increase usability and performance in p2p networks.
Content discovery and recommendations is based on taste buddies, i.e. users with similar interests.
Talks about decentralization/availability/integrity/incentives/network transparency as being fundamental challenges in p2p networks.
Architecturally, it is a set of extensions to Bittorrent. It has a module for social networking, and ‘megacaches’ to locally query metadata. This meetadata are things like friends list (information on social network); peer cache (info on super peers); preference cache (preference list of other peers). All of these are under 10MB in size.
The problem domain is Bitorrent so static files. In UI there’s downloads/friends/files liked and taste buddies.
They have a buddycast algorithm (epidemic based on social graph) and a ‘peer simularity evaluator’.
Bootstraping use random super peer to get more nodes, then use those other ones.
Session boundary problem - using stable peer ids, i.e. keypairs.
Bloom filters for storing and testing set membership for these megacaches. Filtering peers from messages that are known to destinations.
Size of these filters functions of expected connections. Empirical data: Friendster 240 average friends and FB 9100. [FB ~200 median, 340 average]. (This makes sense, since 100 and then 100*100 with some overlap).
To get a bloom filter with 1% false positive rate you need <10 bits per element, so that’s 260 bytes to test and figure out common friends of friends.
Buddycast: Each peer has a number of taste buddies in their megacache with preference list (what content they want) and random peers (i.e. no known preference list). Then every 15s, each peer can either exploit or explore. Exploit = connect to taste buddy, explore = conect random peer. This ratio can be titrated.
A buddycast message also has taste buddies, top 10 preference list and random peers. Buddycast can be disabled for privacy. Peers are listed either by freshness (random peers) or similarity (~overlapping preference lists?).
Briefly talking about BT and rarest-first, tit for tat, and BTSlave using repeaters to increase content replication.
-
Curious, does the megacache concept scale for messages? Assume a message is 1KB, and average user sends 100 messages a day (70 for slack average sent). If you are talking to 100 other people (~200 friends in some constellation of N chats), that’s 10k messages per day or 10 mb per day already. The key thing here is that it’s metadata Tribler is storing, not messages themselves, which (a) allows it to be a cache, cause data integrity not hard requirement (b) constant space, ish (prune preference list at top N).
-
What would happen if we used this on top for most popular/recent content? Still allowing for other means.
This is their academic paper equivalent to Dispersy — Dispersy 0.0.1 documentation - not clear exactly what overlap looks like. I find it interesting that in their docs they call it Distributed Permissions System, but in the paper they emhpasis data dissemination in challenged networks. EDIT: Oh, cause this is about the Bundle Synchronization in particular, not Dispersy or how they use it.
Introduction
Requires minimal assumptions about reliability of communication/network copmonents. Decentralized and pure p2p. Specifically for challenged networks.
What are examples of challenged networks?
Mainly: Vechuilar ad hoc networks (VANET), Delay Tolerant Networks (DTNs).
Additionally: P2P Networks and smartphones.
What’s challenging about VANETs?
Connection windows are small.
What’s challenging about DTNs?
Connections are only possible when network isn’t very well-utilized.
According to Dispersy, what’s challenging about pure p2p networks?
- Churn - node lifetime measured in minutes
- NAT traversal
- Malicious nodes: frequent interactions
In past, these were treated in isolated. For Dispersy, these concerns are combined.
What’s the main idea in Dispersy?
Nodes advertise locally available data in one message, and repeat random interactions lead to quick spreading of data.
NOTE: It appears that the goal is to spread all bundles to all nodes, i.e. a form of full replication. It is also active, since all nodes are spreading all data.
Individual bundles can appear out of order, so it’s different from Delivery in Bramble. They suggest not having dependencies on bundles.
Key things in paper:
- 1:N and M:N data dissemination with minimal complexity/state info
- Easy repeated random interactions between nodes, wrt churn, failure and NATs
- Allow for 100k bundles+ and M+ nodes
- Experimental data and real-world usage
Related work:
What are the three forms of distributing updates Demers proposed?
direct mail, anti-entropy and rumor mongering.
According to Demers, how does direct mail data distribution work?
Update is sent from one node to all other nodes.
According to Demers, how does anti-entropy data distribution work?
Node chooses random node and resolve difference in state.
According to Demers, how does rumor mongering data distribution work?
Node receive new update (hot rumor), then forward update until it goes cold (received from many nodes).
Demers initially thought anti-entropy too expensive; optimiztion to use check-sums etc. (NOTE: Before Bloom filters popular?)
VANET can be seen as P2P due to churn and many nodes w/o central component.
What is the network churn in VANETs due to?
Movement of the vechile.
Lee had urban vechiles with event summary such as license plate and then bloom filter to detect missing summaries. Bloom filters as being very efficient for membership testing, in Lee’s case using 1024 Bytes. (NOTE: for 1% false positive rate that’d be ~1000 cars). They also coupled this with a push to one-hop neighbor. The bloom filter here is thus only used to detect missing summaries. They call this a form of harvesting.
System Overview
‘Bundle synchronization middleware’ for challenged networks. Extends the ant-entropy method using compact hash representation (Bloom filters). Allows two nodes to compare data sets w/o sending complete copy.
TODO: Insert overview of sync and receive
In Dispersy, What does the synchronization five steps look like?
- Node selection
- Bundle selection (some range)
- Bloom Filter creation
- Send BloomFilter (NAT traversal)
- Wait for reply (Pause and goto start)
Synchronization extends harvesting by Lee. They only have a range of bundles, which keeps false positive rate low w/o big bloom filter.
NOTE/QUESTION: How does that follow? Bloom filter size is dteremined by bits-per-element (m/n), but if the element is the set of possible bundles what does it matter what the specific range is? Does this imply the recipient knows which range it is supposed to receive, in order to limit the universe of possible elements?
They say “in contrast Lee used fixed size w/o selecting bundles”. This seems to imply the recipent knows what the range is.
System Components
Allow for different types of challenged networks, so Dispersy is modular. They have a few different components:
- Network Construction
- Node Discovery
- Robust Node Selection
- NAT puncturing
- Synchronization
(- Synchronization performance)
Network construction
All nodes communicate in one or more overlays.
What’s an overlay in p2p?
An additional network build on top of e.g. Internet.
A public key is used as identifier of an overlay, which has to be known to nodes joining.
NOTE: Is this sloppily written or am I misunderstanding? Is this just NodeID or do they mean some kind of bootstrap node / Network ID? EDIT: I guess if we look at each node as having their own overlay.
Why did Dispersy choose to run on UDP over TCP?
Because it allows for better NAT firewall puncturing.
Each overlap has a candidate list,
What is the candidate list that each Dispersy node has in each overlay?
List of active connections within that overlay.
When we connect to an overlay, the candidate list is empty, thus requiring node discovery.
Node Discovery
What’s the most basic form of node discovery if you have an Internet connection?
Using trackers.
What characteristics does a tracker have for node discovery?
(1) Known to all nodes (2) Must be connectable (not behind NAT-firewall).
What does a tracker do in terms of node discovery?
(1) Maintains list of nodes for several overlays (2) Returns these upon request.
After populating candidate list with initial nodes, for example by asking a tracker, these can be used for new connections.
A node A asks node B for introduction to new node, B sends reply with node C. Then A can puncture NAT.
Description of NAT puncture mechanism, more in later section.
Robust Node Selection
We have to select a node from candidate list to send intro request to, but there might be attacker nodes in the list.
Why is node selection in p2p non-trivial?
You might connect to a malicious node, leading to e.g. eclipse attack.
What’s manipulating the candidate list of nodes in p2p called so that it includes attack nodes?
Eclipse attack.
What can a malicious node do after eclipsing a node?
Control all data received by victim.
How does Dispersy create a more robust node selection algorithm?
By dividing candidate list into three categories, one with two subcategories.
What are the 3-4 selection list categories in Dispersy?
- Trusted nodes
- Nodes we contacted before (successfully)
- Nodes that contacted us before
3a) Received introduction request
3b) Nodes introduction
When selecting a node from candidate list, category 1 is 1% of the time, 99% divided by category 2 and 3. Each subcategory gets 24.75% of the time.
NOTE/TODO: This probably generalizes to how Bitcoin and Ethereum deals with Eclipse attacks, in terms of buckets etc. Might be worth comparing these.
For each step in Dispersy node selection, what’s the probability of choosing each category?
1% trusted, ~50% nodes we connected to before, and ~25% for each of other nodes having contacted us before.
How does Dispersy’s node selection algorithm guard against eclipse attacks?
Attacker nodes will (usually!) end up in the third bucket, which has a cap on the selection probability.
In terms of which node is selected in each category, this is elaborated on later. But roughly oldest for NAT timeouts, as well as most similar friend (IIRC).
If an attacker has a lot of resources they can still eclipse node, hence the use of trusted nodes.
NOTE/TODO: Eclipse video worth rewatching with better notes on mitigations. Also: How does 1% impact eclipse possibility to do harm? If you have limited amount of connections you might still not reach 1% case, so seems to depend on what harm individual/set of nodes can do.
Trusted nodes (i.e. trackers): Contacting a trusted node will completely reset candidate list (!). They did experiments with manipulating category 2 nodes.
Why are trusted nodes (trackers) less suspectible to eclipse attacks?
They are contacted a lot and constantly by honest nodes, which makes it harder to pollute the candidate list. (N.B. Sybil attacks).
NOTE/TODO: Read up more on Sybil attack and Sybil resistance.
NOTE: They seem to claim 4 million concurrenct nodes in P2P empirically (cite 18, Gnutella), and implicitly that the risk of Sybil attack for that is low.
NOTE/TODO: How does recency impact the sybil attack resistance? IIRC in Eclipse presentation there’s an argument that old nodes are safer, I guess this depends on bucket design? I.e. if % allocation changes.
NAT puncturing
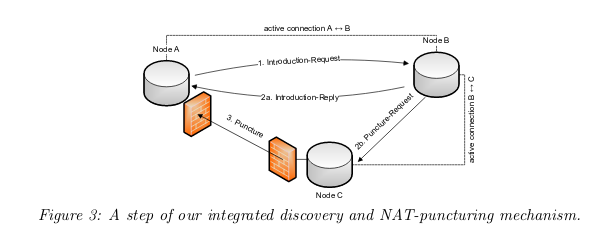
How many nodes connected to the Internet are behind a NAT, according to Halkes et al 2011?
Two thirds, up to 64%.
NOTE/TODO: this number might be even higher nowadays with smartphones? Read up on current state of NAT traversal, and how this changes with different protocols, i.e. QUIC etc.
As a consequence of this, each introduction step is used with distributed UDP NAT puncturing mechanism.
How does Dispersy get around the fact that 64% of nodes are behind a NAT?
By integrating each node discovrey step with a distributed UDP NAT puncturing mechanism.
How does the UDP NAT puncturing mechanism work in Dispersy? Three steps.
- When A asks for introduction B, B sends introduction-reply to A AND performs a puncture-request to C (with A’s info)
- C then sends puncture message to Node A
- C’s message will be blocked by A, but when A selects C they can connect to them
NOTE/TODO: A bit fuzzy on the details of how the ports are matched up, worth revisiting basics. Same re NAT timeouts.
Due to NAT timeouts, nodes are invalidated after some time (~30s-1m). See Halkes (cite 9) for more.
Synchronization
Bundle synchronization through bloom filters, allow nodes to check for missing bundles. Additionally, piggybacking on introduction mechanism to allow just one message to be sent.
Why is it extra important to limit size of bloom filter in Dispersy?
Due to UDP + piggybacking on introduction message, so that the MTU of link isn’t exceeded.
What happens if the MTU of the link is exceeded for UDP?
If UDP consists of multiple fragments, there’s a higher probability it will be lost.
What’s a typical MTU size used on the Internet, according to RFC 3580?
1500 Bytes (2304B for 802.11).
NOTE: Might be more up to date resources for this / gotchas for various links.
NOTE/TODO: How big would e.g. Sphinx packet format be? Same for various types of standard cryptographic payloads, e…g Handshake. If they are written with TCP in mind, perhaps this is a gotcha.
<1000 bundleds can be synchronized with 1% false positive rate for 1024 Bytes; <2000 for 10% false positive rate.
Assuming bloom filter of 1024B, how many messages can we synchronize with 10% false positive rate?
<2000
What’s a flaw in Lee’s MobEyes bloom filter design that uses 1024 Bytes filter?
There’s no limit on bundles being synced, which means the false positive rate shoots up if we add e.g. 2000 vechiles (10% for x2).
How does Dispersy get around the flaw in Lee’s MobEyes bloom filter parameter choices AND MTU limitations?
By fixing false positive rate to 1% as a constant, and limiting number of bits available (MTU) (m), this means Bloomfilter has a fixed capacity, so we select subset of bundles to synchronize (n).
Subset selection
Based on global time property of each bundle, which is an implementeation of Lamport’s logical clock.
What property is subset selection in Dispersy based on?
Global-time property of each bundle, which is using Lamport’s logical clock.
Global time is highest global time received by node + 1 when creating a bundle. NOT unique, but provides partial ordering in overlay. Assume distribution is uniform ish.
Two heuristics for defining which subset to sync.
How does global time property help selecting what to sync in Dispersy?
By specifying a subset range of this (non-unique) global time to sync.
What heuristics are used in Dispersy to select subset to sync in Dispersy and when are they used?
Modulo heuristic when a node is lagging behind, and pivot heuristic when it’s almost up to date.
Modulo heuristic: count number of bundles, divide by Bloom filter capacity. E.g. 10k bundles, bloom filter 1000 capacity, use modulo 10. Then use random offset, e.g. select every 10+n, e.g. 1, 11, 21 etc.
What does modulo heuristic in Dispersy resemble and what does it not require?
Linear download (1, 11, 21 etc) and doesn’t require state.
What’s the downside of modulo heuristic in Dispersy?
If almost caught up, it’d take a modulo number of steps to sync up, e.g. 10th bundle +1/+2 etc offset.
For pivot sync, using exponential distribution between 0 and (locally known global time). Then select bloom filters to left and right, and pick the one~. (NOTE: A bit hazy on exact details here, would need to revisit; exponential means bias towards new).
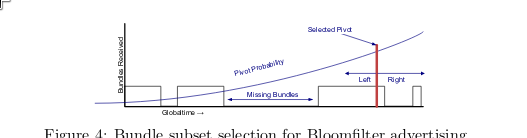
**NOTE/TODO: How would we allow for these types of sync modes? It’d require global-time partial ordering visible for all nodes, AFAIUI
What does a node do when receiving Bloom filter in Dispersy?
Checks if it has missing bundles to send, based on local subset of bundles and testing against Bloom Filter.
What does receiving node in Dispersy need to find out if it has missing bundles to send?
- Bloom filter
- Subset defined by: a) range of global-time b) modulo and c) offset value.
Synchronization performance
Nice math based on false positive rate, MTU, bundles etc. Depending on synchronization level.
NOTE: Interesting to note re false positive rate, AFAIUI this is inversely related to BW perf.
Evaluation and evaluation
Actual simulation and empirical study of things described above. Including NAT experiments, random node selection etc. Skimming for now.
Churn resiliance based on session time. Propagation speed. Bandwidth consumption. High workloads. Internet deployment (integrate into Tribler).
NOTE: Cell phone perfmrance is much worse than WiFI connections. I.e. carrier grade APDM routers, hard to puncture. Also UDP concerns.
NOTE/TODO: Look into smartphone NAT traversal state of the art.
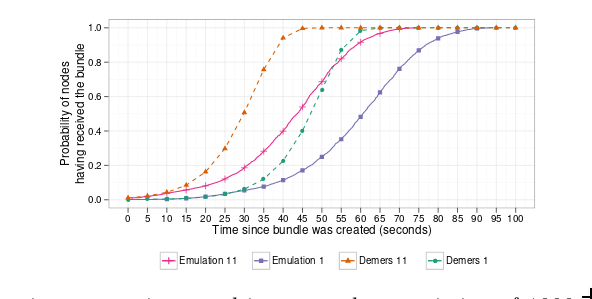
To sync 100k bundles Dispersy connected to ~1000 nodes and consumed 30 Mbytes of BW per run. (NOTE: Assuming bundle is ~1KB that’s 30*1000=30k for 100k or 1/3? Eh, unit is off somewhere).
Sucess rate of 77% for 15 different NAT traversal (N.B. smartphone worse). Can handle excessive churn (session times 30s).
Further reading and conclusion
Awesome paper, much better and clearer than Tribler Dispersy docs. Wish I read it in detail earlier. Lots of beautiful ideas and backed up by real world experience and math. Still need to think about the untrusted stuff, but at least the dependencies and mechanics are more clear.
Some citations from paper:
-
Bloom, Burton H. “Space/time trade-offs in hash coding with allowable errors.” Communications of the ACM 13.7 (1970): 422-426.
-
Demers, Alan, et al. “Epidemic algorithms for replicated database maintenance.” ACM SIGOPS Operating Systems Review 22.1 (1988): 8-32.
-
Halkes, Gertjan, and Johan Pouwelse. “UDP NAT and Firewall Puncturing in the Wild.” International Conference on Research in Networking. Springer, Berlin, Heidelberg, 2011.
-
Huang, Hong-Yu, et al. “Performance evaluation of SUVnet with real-time traffic data.” IEEE Transactions on Vehicular Technology 56.6 (2007): 3381-3396.
-
Laoutaris, Nikolaos, et al. “Delay tolerant bulk data transfers on the internet.” ACM SIGMETRICS Performance Evaluation Review. Vol. 37. No. 1. ACM, 2009.
-
Lee, Uichin, et al. “Dissemination and harvesting of urban data using vehicular sensing platforms.” IEEE transactions on vehicular technology 58.2 (2009): 882-901.
-
Singh, Atul, et al. “Defending against eclipse attacks on overlay networks.” Proceedings of the 11th workshop on ACM SIGOPS European workshop. ACM, 2004.
Ran out of time for timebox, and still have some synthesizing to do, but thought I’d post it here for e.g. Dean et al. TODO: understand in more detail the following points:
- What exactly would need to be added to our current setup to enable this (e.g. transparent global time and subset indicators)
- How this compares with current/Briar ish approach wrt untrusted node assumptions and latency (sync delay quite big) - how can we get best of both worlds? Also bloom filter privacy.